Sigma rules for Linux and MacOS
Welcome macOS and Linux
logsource:
product: linux
category: process_creation
detection:
selection:
Image|endswith: ‘/crontab’
CommandLine|contains: ‘ -l’
condition: selection
Linux, MacOS and Windows examples
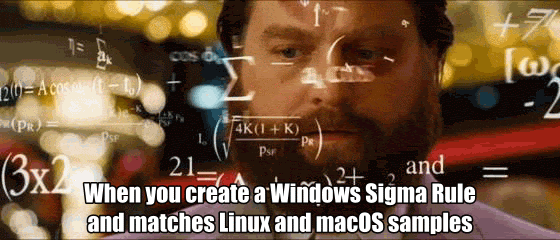
Creating Livehunt rules from Sysmon EVTX outputs
import
“vt”
rule
sigma_example_registry_keys {
meta:
target_entity
= “file”
condition:
for
any
vt_behaviour_sigma_analysis_results in
vt.behaviour.sigma_analysis_results: (
for
any
vt_behaviour_sigma_analysis_results_match_context in
vt_behaviour_sigma_analysis_results.match_context: (
vt_behaviour_sigma_analysis_results_match_context.values[“TargetObject”]
icontains
“\\CurrentVersion\\RunOnce\\”
and
(vt_behaviour_sigma_analysis_results_match_context.values[“Details”]
endswith
“.vbs”
or
vt_behaviour_sigma_analysis_results_match_context.values[“Details”]
endswith
“.bat”)
)
)
}
141e87e62c110b86cf7b01a2def60faab6365f6391eb0d4a7cbad8d480dd4706
814b2cab7c5a12ec18f345eb743857e74f5be45c35642dc01330e7a0def6269a
31b0e9b188fe944d58867bbfc827d77c7711c3a690168a417377fe6bf1544408
dd6051509ed8cf3d059b538fa8878f87423c51b297b49a12144d3d2923c89cce
647323f0245da631cef57d9ca1e3327c3242fe1cbbf6582c4d187e9f5fbfb678
40a90dd3b2132a299f725e91a5d0127013b21af24074afb944d8bc5735c1bd53
b44c6d2dd8ad93cecd795cecde83081292ee9949d65b2e98d4a2a3c8a97bd936
710b0cca7e7c17a3dd2a309f5ca417b76429feac1ab5fb60f5502995ebbd1515
50c098119ce41771e7a3b8230a7aa61ebea925e8eda46c33f0dd42b8950b92fe
import
“vt”
rule
sigma_rule_evtx_cve {
meta:
target_entity
= “file”
condition:
for
any
vt_behaviour_sigma_analysis_results in
vt.behaviour.sigma_analysis_results: (
for
any
vt_behaviour_sigma_analysis_results_match_context in
vt_behaviour_sigma_analysis_results.match_context: (
vt_behaviour_sigma_analysis_results_match_context.values[“TargetFilename”]
startswith
“C:\\Windows\\System32\\”
and
vt_behaviour_sigma_analysis_results_match_context.values[“TargetFilename”]
endswith
“.dll”
and
for
any
vt_metadata_tags in
vt.metadata.tags: (
vt_metadata_tags
icontains
“cve-“
)
)
)
}
Sysmon EVTX fields – overlaps
vt_behaviour_sigma_analysis_results_match_context.values[“TargetFilename”] from vt.behaviour.sigma_analysis_results
- Sysmon information is fully stored/indexed only the part matching the Sigma rule, which will limit any YARA hunting.
- We mapped most Sysmon fields into YARA VT module for simplicity.
- Linux and MacOS samples do not have any Sysmon information related to Sigma rules. Similar details about the match can be found under the “behaviour” JSON structure entry.
VT |
YARA |
Sigma |
Sigma |
behavior_created_processes |
vt.behaviour.processes_created |
process_creation |
Image CommandLine ParentCommandLine ParentImage OriginalFileName |
behavior_files |
vt.behaviour.files_attribute_changed vt.behaviour.files_deleted vt.behaviour.files_opened vt.behaviour.files_copied vt.behaviour.files_copied[x].destination vt.behaviour.files_copied[x].source vt.behaviour.files_written vt.behaviour.files_dropped vt.behaviour.files_dropped[x].path vt.behaviour.files_dropped[x].sha256 vt.behaviour.files_dropped[x].type |
file_access file_change file_delete file_rename file_event |
TargetFilename |
behavior_injected_processes |
vt.behaviour.processes_injected |
process_access create_remote_thread process_creation |
CallTrace GrantedAccess SourceImage TargetImage StartModule StartFunction TargetImage SourceImage |
behavior_processes |
vt.behaviour.processes_terminated vt.behaviour.processes_killed vt.behaviour.processes_created vt.behaviour.command_executions vt.behaviour.processes_injected |
process_access create_remote_thread process_creation |
CallTrace GrantedAccess SourceImage TargetImage StartModule StartFunction TargetImage SourceImage Image CommandLine ParentCommandLine ParentImage OriginalFileName |
behavior_registry |
vt.behaviour.registry_keys_deleted vt.behaviour.registry_keys_opened vt.behaviour.registry_keys_set vt.behaviour.registry_keys_set[x].key vt.behaviour.registry_keys_set[x].value |
registry_add registry_delete registry_event registry_rename registry_set |
EventType TargetObject Details |
behavior_services |
vt.behaviour.services_bound vt.behaviour.services_created vt.behaviour.services_opened vt.behaviour.services_started vt.behaviour.services_stopped vt.behaviour.services_deleted |
registry_set process_creation |
Image CommandLine ParentCommandLine ParentImage EventType TargetObject Details |
behavior_network |
vt.behaviour.dns_lookups vt.behaviour.dns_lookups[x].hostname vt.behaviour.dns_lookups[x].resolved_ips vt.behaviour.hosts_file vt.behaviour.ip_traffic vt.behaviour.ip_traffic[x].destination_ip vt.behaviour.ip_traffic[x].destination_port vt.behaviour.ip_traffic[x].transport_layer_protocol vt.behaviour.http_conversations vt.behaviour.http_conversations[x].url vt.behaviour.http_conversations[x].request_method vt.behaviour.http_conversations[x].request_headers vt.behaviour.http_conversations[x].response_headers vt.behaviour.http_conversations[x].response_status_code vt.behaviour.http_conversations[x].response_body_filetype vt.behaviour.smtp_conversations[x].hostname vt.behaviour.smtp_conversations[x].destination_ip vt.behaviour.smtp_conversations[x].destination_port vt.behaviour.smtp_conversations[x].smtp_from vt.behaviour.smtp_conversations[x].smtp_to vt.behaviour.smtp_conversations[x].message_from vt.behaviour.smtp_conversations[x].message_to vt.behaviour.smtp_conversations[x].message_cc vt.behaviour.smtp_conversations[x].message_bcc vt.behaviour.smtp_conversations[x].timestamp vt.behaviour.smtp_conversations[x].subject vt.behaviour.smtp_conversations[x].html_body vt.behaviour.smtp_conversations[x].txt_body vt.behaviour.smtp_conversations[x].x_mailer vt.behaviour.tls |
network_connection |
DestinationHostname DestinationIp DestinationIsIpv6 DestinationPort DestinationPortName SourceIp SourceIsIpv6 SourcePort SourcePortName |
behavior (too generic) |
vt.behaviour.modules_loaded |
image_load |
ImageLoaded Image OriginalFileName |